Excerpts from the report…
Most recommendation engines are based on collaborative filtering, which looks at the affinity between two products in the same category or between two categories. It has limitations because it only looks at past purchase history of a consumer. So if a consumer bought a striped shirt or searched for it, she will keep getting recommendations even if she has closed that deal. So just using internal data doesn’t work. “To make the right kind of guided discovery, one must combine internal data generated inside the company with external data,” says Srikant Sastri, co-founder, Crayon Data. “External data can be generated by trailing the internet reviews and social networking for movies, books, shopping, travel etc. Building cross-category connection on the basis of external and internal data can make recommendations richer and powerful.” [Read More]
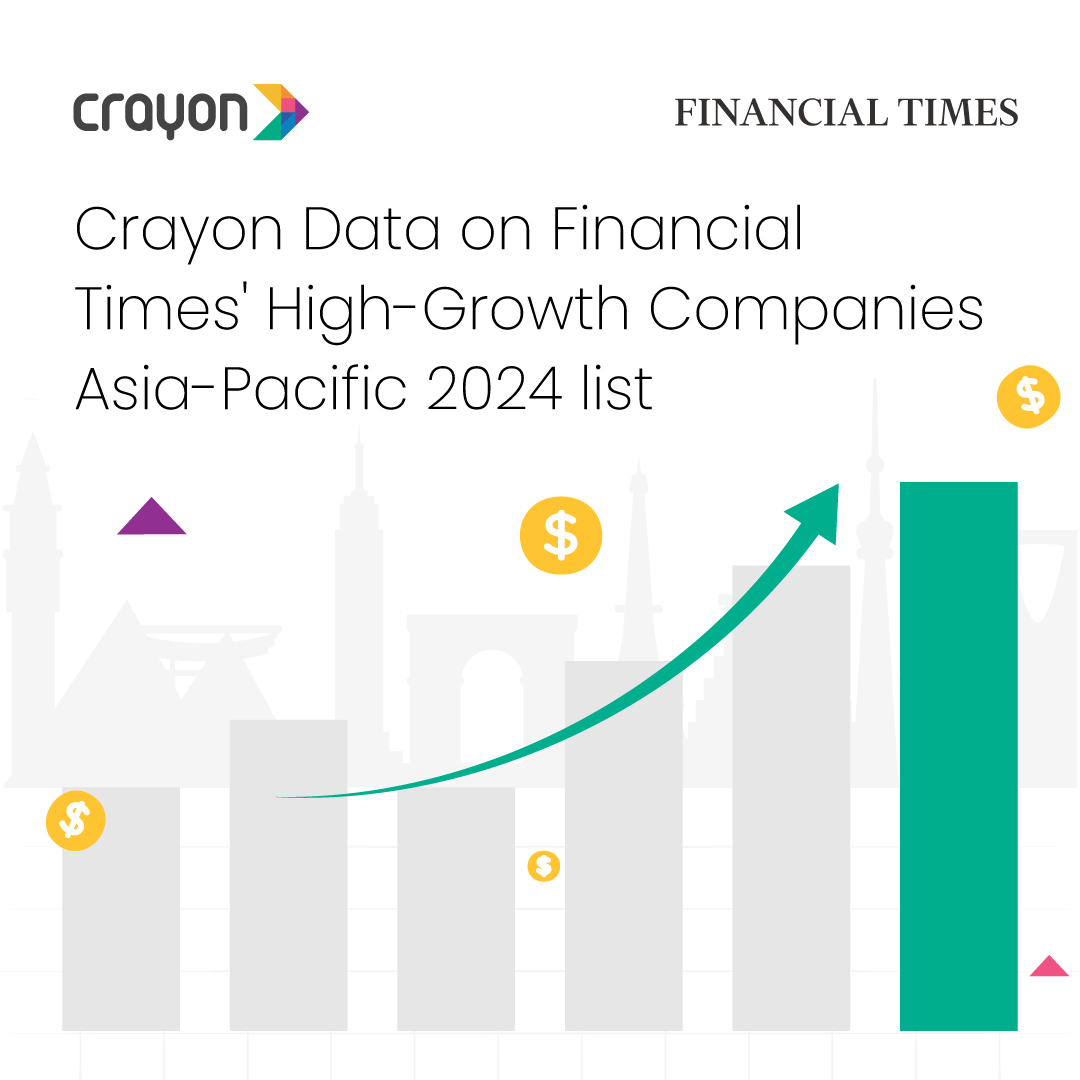
Crayon Data on Financial Times ‘ High-Growth Companies Asia-Pacific 2024 list
Date:...