Product recommendations for ecommerce brands
July 9, 2023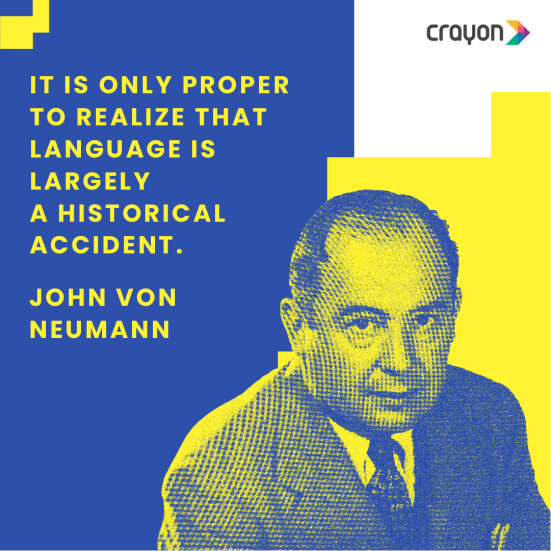
Legends of AI: John Von Neumann
July 14, 2023#TheAIAlphabet
A for Attention
Published April 10, 2024 | Sunantha Sanjeeva Rao
The Attention mechanism is a significant breakthrough that makes Generative AI possible.
Multi-head attention, which is Attention’s current avatar is the force behind all the Transformer models powering the LLMs out there. While it is theoretically possible to develop Generative AI without Attention mechanisms, their absence would have limited the speed of evolution of these models by half a decade at least.
Multi-head attention, which is Attention’s current avatar is the force behind all the Transformer models powering the LLMs out there. While it is theoretically possible to develop Generative AI without Attention mechanisms, their absence would have limited the speed of evolution of these models by half a decade at least.
But, what is Attention?
Attention is like a spotlight. It allows an artificial intelligence (AI) model to focus on the most important parts of an input, whether it’s a sentence, an image, or a piece of music.
Why is this focus required? Such highlighting helps an AI recognize the underlying patterns and relationships more efficiently, thereby improving the model’s performance. It’s like having a secret decoder ring that extracts the juicy secrets hidden in the data, making sense of the jumble.
Imagine you’re reading a book in a noisy room. You can’t process the words very well, so you have to focus on the important parts of the sentence. You might focus on the nouns and verbs, or on the words that are related to the topic of the sentence. That’s what attention is about.
It works by assigning weights to different parts of the data. It’s like giving a rating to each puzzle piece based on how important it is. The pieces with high ratings get the most attention, while the ones with low ratings are get to bask in the background.
This mechanism’s potential is especially useful for models that try to figure out the relationships between different parts of an input.
Quite the dream partner, isn’t it?
Why is this focus required? Such highlighting helps an AI recognize the underlying patterns and relationships more efficiently, thereby improving the model’s performance. It’s like having a secret decoder ring that extracts the juicy secrets hidden in the data, making sense of the jumble.
Imagine you’re reading a book in a noisy room. You can’t process the words very well, so you have to focus on the important parts of the sentence. You might focus on the nouns and verbs, or on the words that are related to the topic of the sentence. That’s what attention is about.
It works by assigning weights to different parts of the data. It’s like giving a rating to each puzzle piece based on how important it is. The pieces with high ratings get the most attention, while the ones with low ratings are get to bask in the background.
This mechanism’s potential is especially useful for models that try to figure out the relationships between different parts of an input.
Quite the dream partner, isn’t it?
Recent Blogs
Subscribe to the Crayon Blog. Get the latest posts in your inbox!