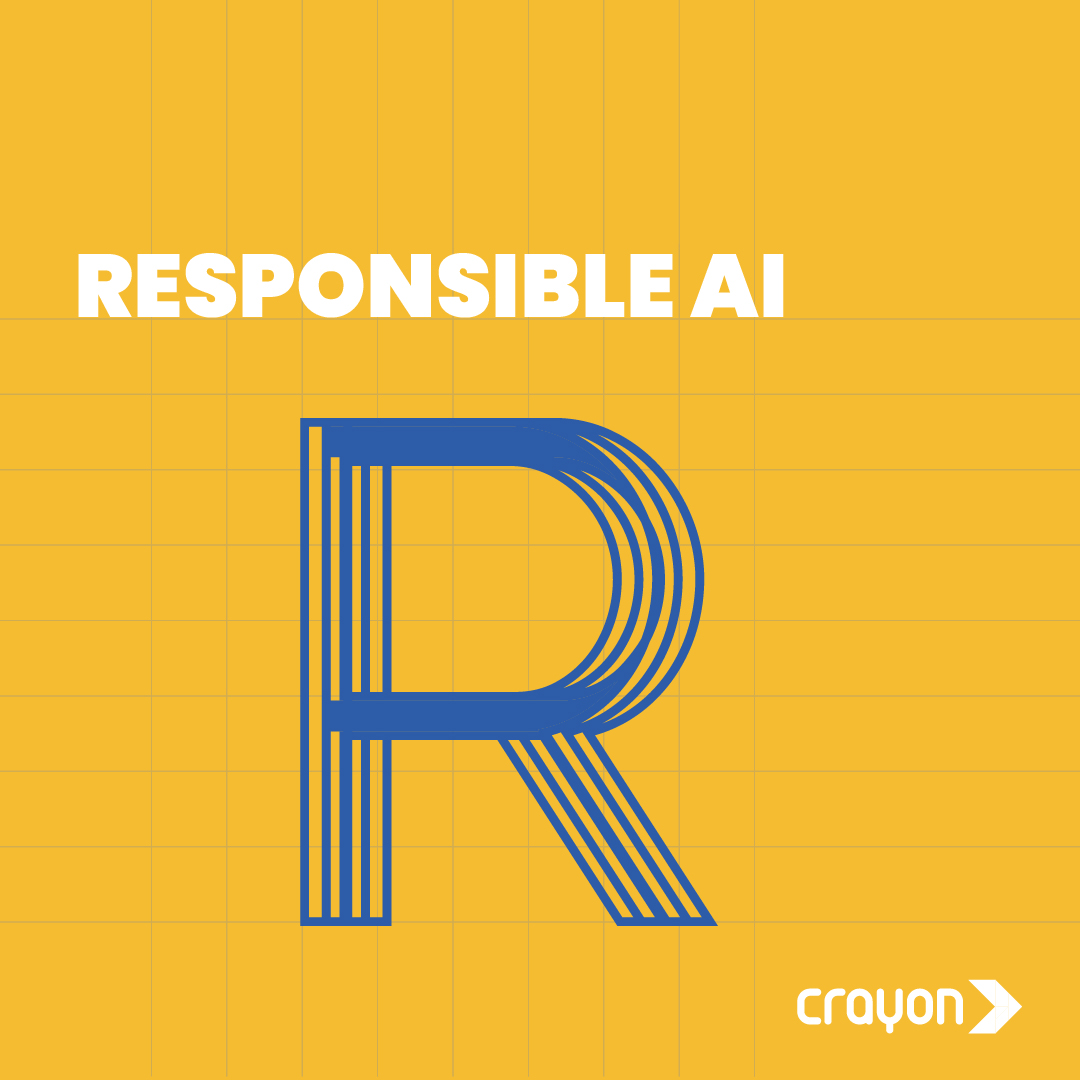
Responsible AI
November 9, 2023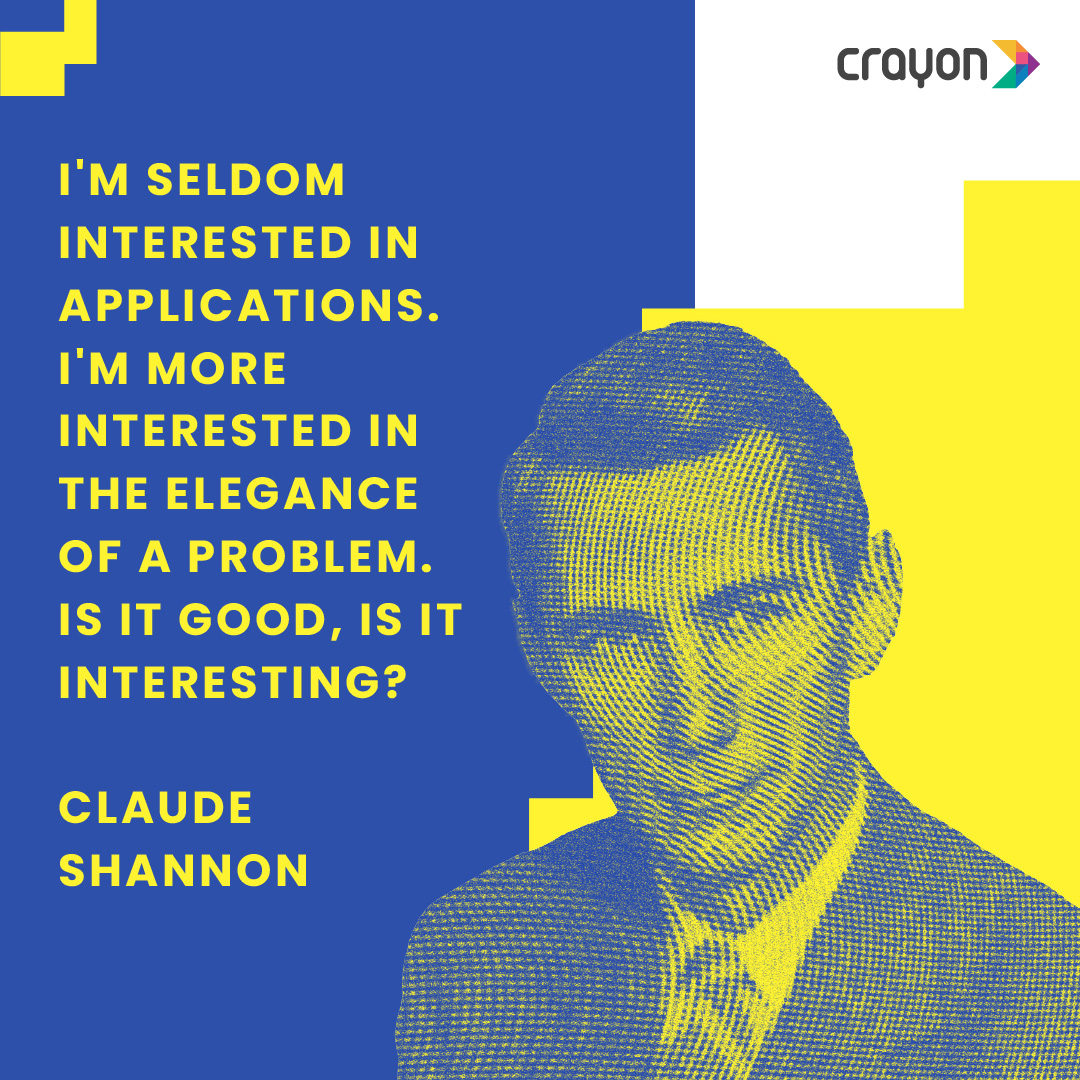
Legends of AI: Claude Shannon
November 17, 2023#TheAIAlphabet
S for Stochastic Parrots
Published November 16, 2023 | Team Crayon
Imagine a parrot that can mimic human speech perfectly, but has no idea what it’s saying. That’s the idea behind a “stochastic parrot,” a term coined by researchers to describe large language models (LLMs) that are good at generating human-like text, but don’t actually understand the meaning of it.
LLMs are trained on massive amounts of text data, and they learn to predict the next word or phrase in a sequence based on the words that have come before. This allows them to generate seemingly fluent and coherent text, even if they don’t understand the meaning of the words they’re using.
LLMs are trained on massive amounts of text data, and they learn to predict the next word or phrase in a sequence based on the words that have come before. This allows them to generate seemingly fluent and coherent text, even if they don’t understand the meaning of the words they’re using.
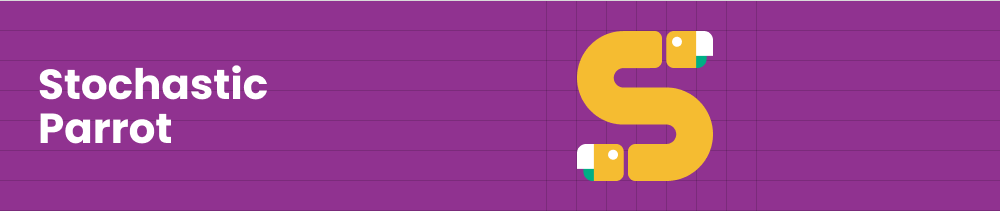
Stochastic parrots can be dangerous because they can be used to generate fake news, propaganda, and other forms of misinformation. They can also be used to impersonate real people, which could lead to identity theft or other forms of harm.
Some researchers argue that the term “stochastic parrot” is unfair to LLMs, because it implies that they are nothing more than copycats. They point out that LLMs can actually learn to perform a variety of tasks, such as translation, summarization, and question answering.
However, others argue that the term is accurate, because LLMs still do not have a deep understanding of language. They can generate text that is grammatically correct and semantically plausible, but they often lack the ability to understand the nuances of meaning.
Despite the debate over the term, it is clear that stochastic parrots pose a number of risks. As LLMs become more powerful, it is important to be aware of these risks and to take steps to mitigate them.
One way to do this is to develop methods for detecting fake news and other forms of misinformation generated by LLMs. Another way is to educate people about the limitations of LLMs, so that they are less likely to be fooled by them.
It is also important to develop responsible AI practices that ensure that LLMs are used in a safe and ethical way. This includes developing guidelines for the development and use of LLMs, as well as training people about the risks of these technologies.
In conclusion, stochastic parrots are a complex and important issue in the field of AI. It is important to be aware of the risks they pose and to take steps to mitigate them. By doing so, we can ensure that LLMs are used in a safe, ethical, and responsible way.
Some researchers argue that the term “stochastic parrot” is unfair to LLMs, because it implies that they are nothing more than copycats. They point out that LLMs can actually learn to perform a variety of tasks, such as translation, summarization, and question answering.
However, others argue that the term is accurate, because LLMs still do not have a deep understanding of language. They can generate text that is grammatically correct and semantically plausible, but they often lack the ability to understand the nuances of meaning.
Despite the debate over the term, it is clear that stochastic parrots pose a number of risks. As LLMs become more powerful, it is important to be aware of these risks and to take steps to mitigate them.
One way to do this is to develop methods for detecting fake news and other forms of misinformation generated by LLMs. Another way is to educate people about the limitations of LLMs, so that they are less likely to be fooled by them.
It is also important to develop responsible AI practices that ensure that LLMs are used in a safe and ethical way. This includes developing guidelines for the development and use of LLMs, as well as training people about the risks of these technologies.
In conclusion, stochastic parrots are a complex and important issue in the field of AI. It is important to be aware of the risks they pose and to take steps to mitigate them. By doing so, we can ensure that LLMs are used in a safe, ethical, and responsible way.
Image recognition: Neurosymbolic learning can be used to improve the accuracy and robustness of image recognition systems. It could be trained to identify cats in pictures, even if the pictures are noisy or contain other animals.
Natural language processing: It can be used to improve the performance of machine translation and question answering. For example, it could be used to translate a text from one language to another, even if the text contains complex grammar or idiomatic expressions.
Reasoning and planning: It can develop AI systems that can reason and plan effectively. For instance, a robot that can navigate its way through a complex environment.
In essence, it’s like teaching AI to think with both its heart (neural networks) and its head (symbolic reasoning), which makes it much more capable and, well, sandwich-savvy in the world of artificial intelligence!
Natural language processing: It can be used to improve the performance of machine translation and question answering. For example, it could be used to translate a text from one language to another, even if the text contains complex grammar or idiomatic expressions.
Reasoning and planning: It can develop AI systems that can reason and plan effectively. For instance, a robot that can navigate its way through a complex environment.
In essence, it’s like teaching AI to think with both its heart (neural networks) and its head (symbolic reasoning), which makes it much more capable and, well, sandwich-savvy in the world of artificial intelligence!
Recent Blogs
Subscribe to the Crayon Blog. Get the latest posts in your inbox!