September 4, 2024
The Crayon Blog
Navigating the Future of Lending: How AI is Revolutionizing Consumer Credit
| Published May 23, 2024 | Sunantha Sanjeeva Rao
Nightmares in increasing order of scariness:
Ghost, non-refundable tickets, credit scores!
As a joke that’s as old as credit scoring goes, “Gets tax return, pays bills and debts on time.
Credit score drops by 100 points.”
At the center of such jokes is a irrefutable reality that credit is still not everyone’s game. Why not? And what can we do to make it a universally simple concept? That’s what this blog is about to tell you.
As a joke that’s as old as credit scoring goes, “Gets tax return, pays bills and debts on time.
Credit score drops by 100 points.”
At the center of such jokes is a irrefutable reality that credit is still not everyone’s game. Why not? And what can we do to make it a universally simple concept? That’s what this blog is about to tell you.
The current state of consumer credit
A study by economists Laura Blattner at Stanford University and Scott Nelson at the University of Chicago show that differences in mortgage approval between minority and majority groups is not just down to bias, but to the fact that minority and low-income groups have less data in their credit histories.
Even for those who have a good grip on finances, the credit getting game may be closer to a gamble than to a process. This skew is a major factor in inaccessibility to financial and entrepreneurial support. How can AI come to the rescue of these woes? Before we get to that, let’s zero in on the traditional lending practice and the challenges that come with it.
Let’s start with the first step in lending – checking credit scores. Traditional way of credit scoring sources data from bank-based credit data and relevant information from publicly available records – information relating to loans or credit limits, repayment of debt, and inquiries into credit history.
While this seems reliable at a textbook level, this approach may overlook the other layers there are to a person’s credibility.
Even for those who have a good grip on finances, the credit getting game may be closer to a gamble than to a process. This skew is a major factor in inaccessibility to financial and entrepreneurial support. How can AI come to the rescue of these woes? Before we get to that, let’s zero in on the traditional lending practice and the challenges that come with it.
Let’s start with the first step in lending – checking credit scores. Traditional way of credit scoring sources data from bank-based credit data and relevant information from publicly available records – information relating to loans or credit limits, repayment of debt, and inquiries into credit history.
While this seems reliable at a textbook level, this approach may overlook the other layers there are to a person’s credibility.
What other layers?
Imagine a person on a tight income – expense cycle. This person wants to open a business and is seeking a loan to fund the business capital. Such a person may not be a bank’s first choice for a loan because of their income level or less savings. So, banks may reject the application despite the following factors:
– The person has a novel, solid business plan / model
– The person has not defaulted on any of his regular bills – phone, insurance, tax, etc.
In addition to these, past applications rejected by banks also paint a red tint on the application.
Seems like a catch-22 situation, right? Less income, no loan. No loan, no betterment in income. And the effect of these scores reaches far beyond access to credit. They are also used by potential employers and landlords to gauge a prospective candidate or tenant, as is the case.
A reform in credit scoring could win the world much more than a booming loan industry. But what is the solution? Bring in AI and Alternate data.
– The person has a novel, solid business plan / model
– The person has not defaulted on any of his regular bills – phone, insurance, tax, etc.
In addition to these, past applications rejected by banks also paint a red tint on the application.
Seems like a catch-22 situation, right? Less income, no loan. No loan, no betterment in income. And the effect of these scores reaches far beyond access to credit. They are also used by potential employers and landlords to gauge a prospective candidate or tenant, as is the case.
A reform in credit scoring could win the world much more than a booming loan industry. But what is the solution? Bring in AI and Alternate data.
The need for change
For those who say, “The current system works fine” – It does not work fine.
1. Hard credit checks hurt credit scores:
There are 2 types of credit checks – hard and soft. While hard credit check is used for the purpose of issuing credit, soft credit check is used for any purpose except credit issuance. Applying for a loan, or credit card, or applying for a rental, phone and utilities can attract a hard credit inquiry. This may cause a noticeable decrease in credit scores.
2. Inaccessibility
Using a hard-and-fast approach to the source of credit scores impedes those from historically disadvantaged backgrounds and those with insufficient or no data on their records, from accessing consumer credit.
3. Restrained loan-shopping
While seeking loans, any sensible person would want to shop around to know the best possible rates and offers. If done within a specific period, credit scoring models rightly gauge multiple hard inquiries as one loan request. But if one continues to go loan-shopping beyond the given period, the hard inquiries might be treated as separate, even though they were intended for the same single loan.
Transforming the scene with AI and alternate data
With a careful use of AI and alternate data, the BFSI sector is improving the scene of credit lending on both the borrowers’ front and lenders’ front.
For the borrowers
1. Reduced mislabeling
America aims to increase black home-ownership to 60% by 2040. But with mislabeling of ethnicity, deserving candidates are denied the promised financial assistance, thereby diluting the goal’s timeline loan.
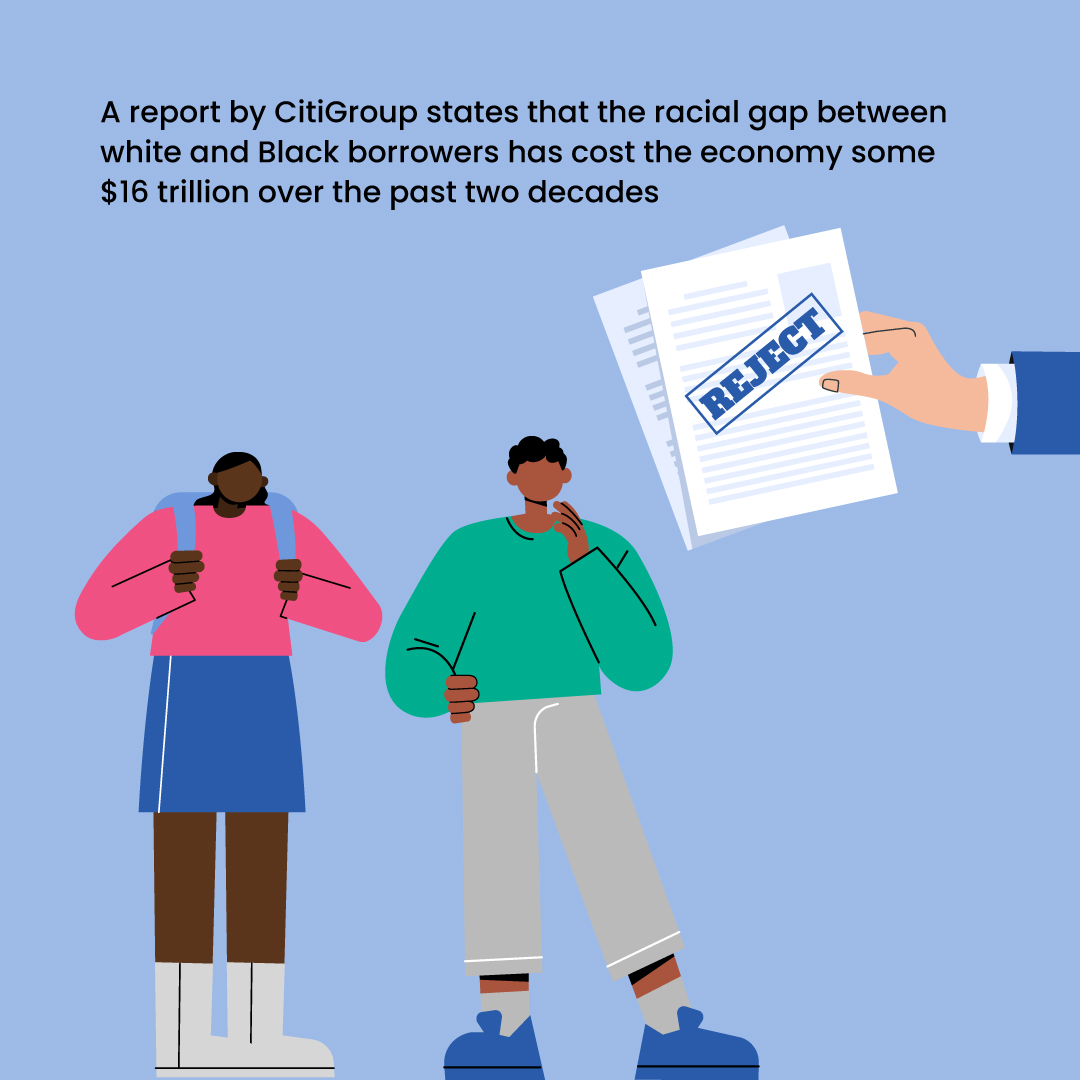
Zest.ai came up with a simple fix that would have an immediate impact on the Black homeownership rate — improve how race is estimated in lending.
But how is this possible when lenders cannot ask the race, be it in person, or through the application? The Bayesian Improved Surname Geocoding (BISG) was invented 2 decades ago to bring fairness in lending. This formula that combines last name and ZIP code, or Census tract, to calculate the best estimate was invented to trace ethnicity, so that the communities that required a taller pedestal to rise to the standard living could be given one.
But as the population got more diverse and denser, the formula that was right 9 out of 10 times in identifying people as Black, especially in racially homogenous areas, correctly identified only 39 percent of Black Americans.
To correct this situation, Zest.ai built Zest Race Predictor, a proposed replacement for BISG. This new model used first, middle, and last names and a richer location data set to calculate the ethnicity. The result:
But how is this possible when lenders cannot ask the race, be it in person, or through the application? The Bayesian Improved Surname Geocoding (BISG) was invented 2 decades ago to bring fairness in lending. This formula that combines last name and ZIP code, or Census tract, to calculate the best estimate was invented to trace ethnicity, so that the communities that required a taller pedestal to rise to the standard living could be given one.
But as the population got more diverse and denser, the formula that was right 9 out of 10 times in identifying people as Black, especially in racially homogenous areas, correctly identified only 39 percent of Black Americans.
To correct this situation, Zest.ai built Zest Race Predictor, a proposed replacement for BISG. This new model used first, middle, and last names and a richer location data set to calculate the ethnicity. The result:
- 23% more accuracy in identifying Black borrowers, when assigning race from the highest probability
- The numbers of whites identified as non-white went down by 61%
2. Smooth dispute redressal
Conversational AI is quickly becoming the new front desk and customer support of enterprises. So, it is only wise that the BFSI industry takes advantage of this feature that simplifies usage for customers.
Credit scores are rarely in the range we think it to be. Therefore, it is one of the most disputed aspects. This dispute redressal process that took days of sending back-and-forth responses, can now be reduced to a matter of minutes with an interactive chat, thanks to conversational AI.
Not just dispute redressal, conversational AI can also notify customers about important changes on their credit report, in real-time. This can reduce the number of complaints raised and help combat identity theft and fraud.
Credit scores are rarely in the range we think it to be. Therefore, it is one of the most disputed aspects. This dispute redressal process that took days of sending back-and-forth responses, can now be reduced to a matter of minutes with an interactive chat, thanks to conversational AI.
Not just dispute redressal, conversational AI can also notify customers about important changes on their credit report, in real-time. This can reduce the number of complaints raised and help combat identity theft and fraud.
3. Improved Financial inclusion
It is not rare to hear stories of people who were denied a loan because of inadequate data on their records. We now have a chance to make these stories less common by bringing in alternative data.
Nigeria is a country with strong entrepreneurial element and immense opportunities. With an estimated 37 million micro, small and medium-sized companies, it poses strong potential, limited only by access to financial services. When Covid-19 struck,
Nigeria is a country with strong entrepreneurial element and immense opportunities. With an estimated 37 million micro, small and medium-sized companies, it poses strong potential, limited only by access to financial services. When Covid-19 struck,
- Many were deprived of an income. This meant that even the worthiest of the borrowers may not have it written on their credit score
- 27% of Nigeria’s labor force (over 21 million Nigerians) were unemployed
LendMe, an Africa based lender, used LenddoEFL’s credit scoring model for nano-loans. This model uses alternative data like behavior and personality traits to predict default risk.
By combining a tighter credit policy and credit risk models built with a focus on long-term stability in addition to predictive power, LendMe has been able to continue lending to customers needing credit without taking unnecessary risk.
LenddoEFL VP Corporate Development, Camille O’Sullivan, said, “For us, COVID19 has shone a light on the fact that, while your financial situation may change, your core character traits tend to be much more stable. By looking at a customer’s interests (through the apps they download), their reliability, perfectionism or stability traits we are able to provide a more complete profile of an individual.”
By combining a tighter credit policy and credit risk models built with a focus on long-term stability in addition to predictive power, LendMe has been able to continue lending to customers needing credit without taking unnecessary risk.
LenddoEFL VP Corporate Development, Camille O’Sullivan, said, “For us, COVID19 has shone a light on the fact that, while your financial situation may change, your core character traits tend to be much more stable. By looking at a customer’s interests (through the apps they download), their reliability, perfectionism or stability traits we are able to provide a more complete profile of an individual.”
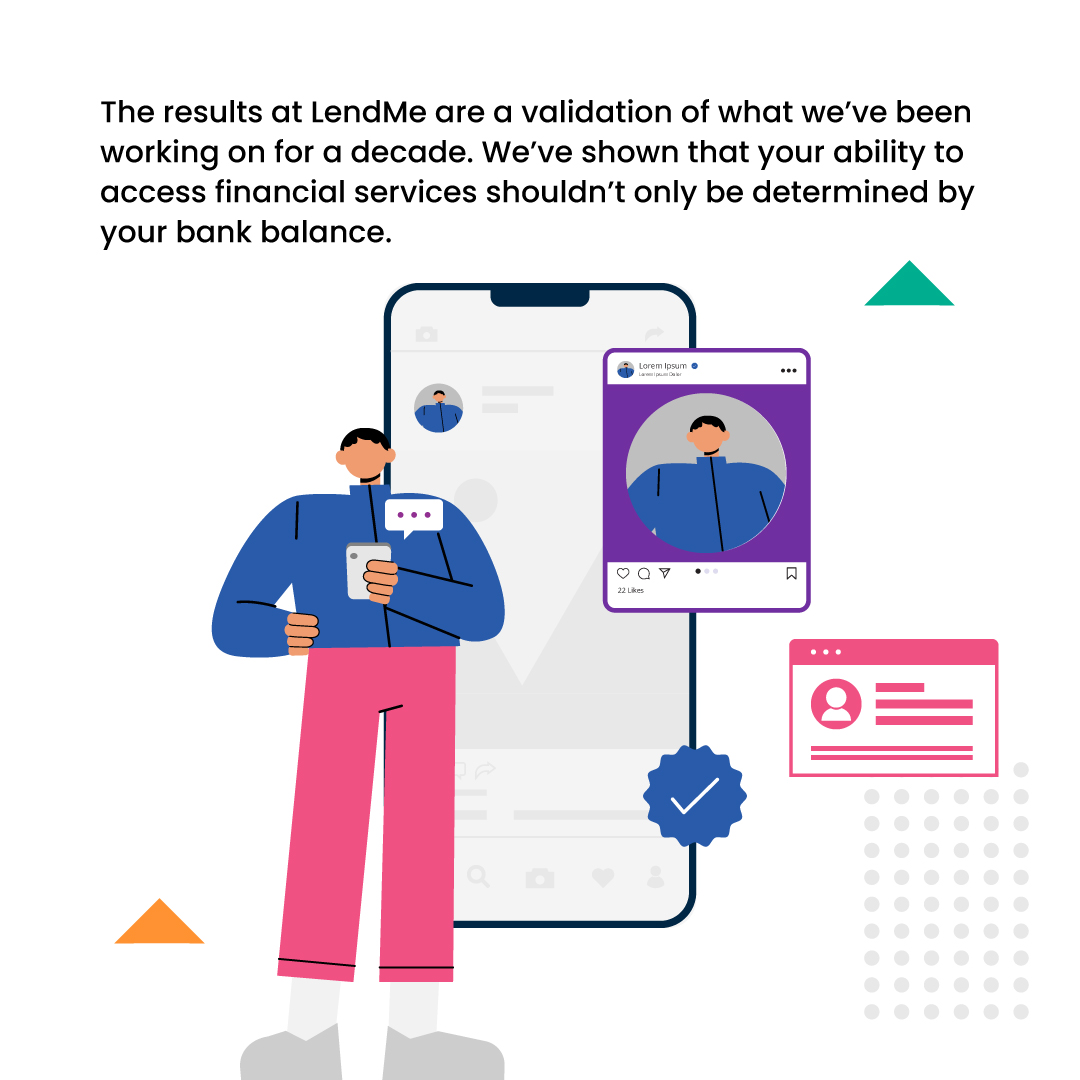
The Women Entrepreneurship Development Project (WEDP) launched by the Government of Ethiopia is another wonderful case study for how alternate methods of credit scoring can foster financial inclusion.
In 2015, Amhara Credit and Savings institution, the largest MFI in the country with over 1 million active borrowers and 440 branches, collaborated with LenddoEFL to use psychometric testing to assess credit risk of the applicants.
In 2015, Amhara Credit and Savings institution, the largest MFI in the country with over 1 million active borrowers and 440 branches, collaborated with LenddoEFL to use psychometric testing to assess credit risk of the applicants.
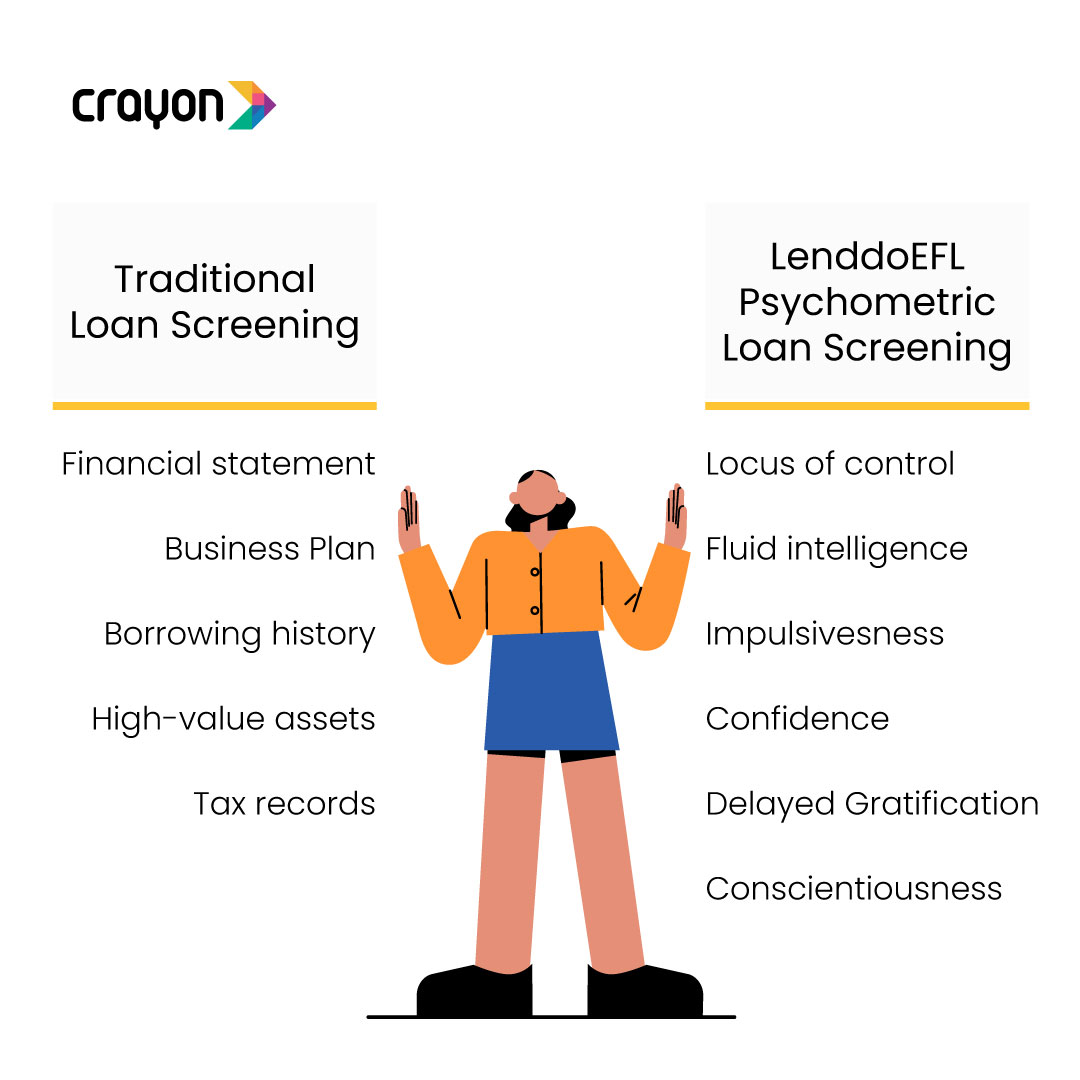
The tests included more visuals and interactive exercises, keeping in mind the low literacy rate and low tech usage. The pre-pilot covered 420 interested clients in 2 branches, and the pilot was conducted across 12 branches with 2496 clients.
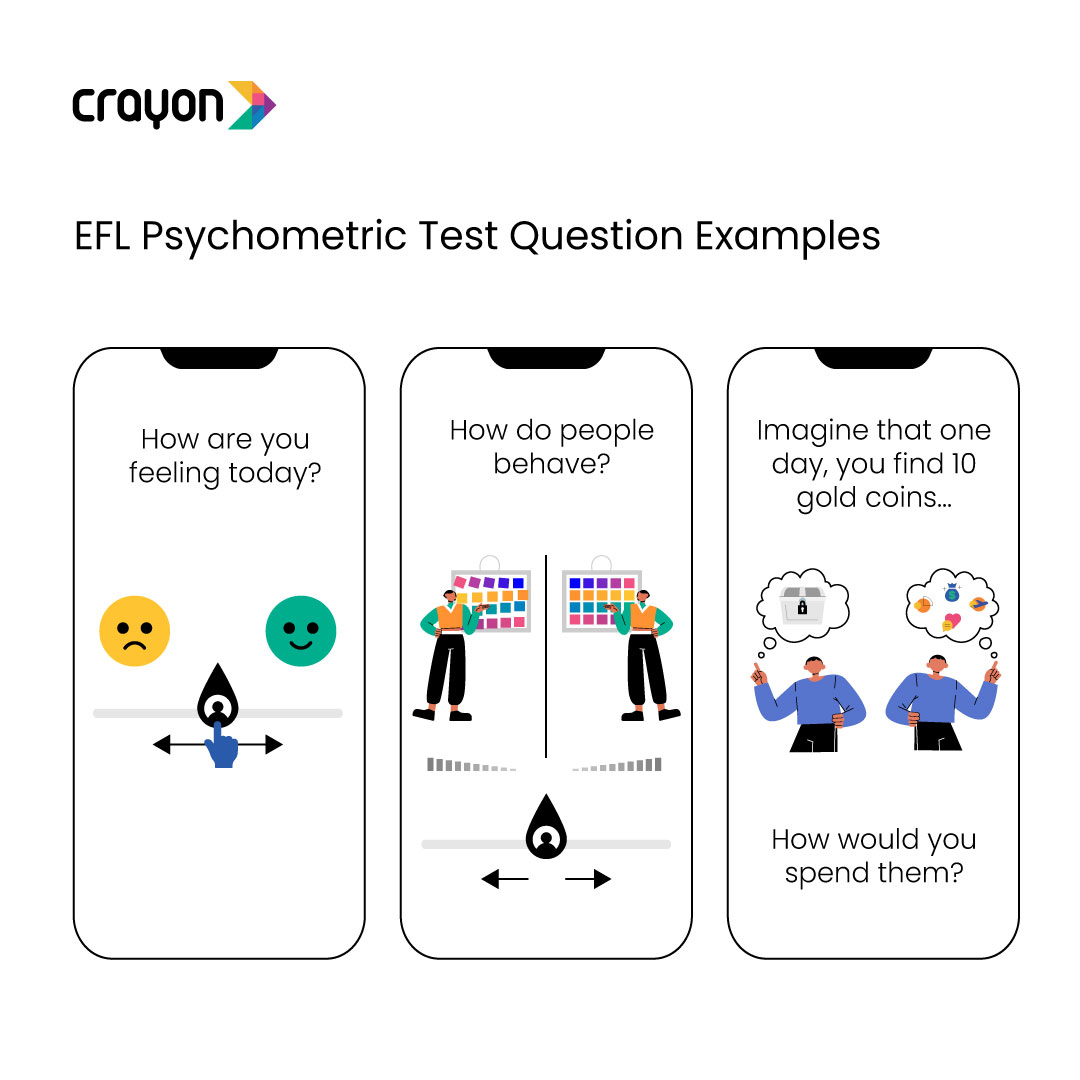
Post loan disbursement, the data showed a clear connection between the test scores and loan performance. Borrowers who scored higher on the test were 7X more likely to repay their loans than those who scored less.
For the frauders
1. Fraud detection
AI can help trace the creditworthiness of an applicant and run checks for any history of fraud or loan stacking or default, thus minimizing risk for the lender.
Also, it is not possible for humans to keep a perpetual watch on borrowers’ data. Here’s where AI becomes an invaluable tool – along with active monitoring round the clock, it can flag suspicious activities and warn the lender at the earliest.
Also, it is not possible for humans to keep a perpetual watch on borrowers’ data. Here’s where AI becomes an invaluable tool – along with active monitoring round the clock, it can flag suspicious activities and warn the lender at the earliest.
2. Increased efficiency
Evaluating an application has been a task that takes up a few days. With AI on your side, this process can now be a matter of few hours. Not just application evaluation, automation of communications and customer support can also enable lenders operate more efficiently.
A leading Buy-Now-Pay-Later (BNPL) platform with a user base of 10 million+ wanted to streamline their collection operation better. A fast-growing user base warranted a more tailored and robust approach. Their current system had the following drawbacks.
A leading Buy-Now-Pay-Later (BNPL) platform with a user base of 10 million+ wanted to streamline their collection operation better. A fast-growing user base warranted a more tailored and robust approach. Their current system had the following drawbacks.
– Manual calling was not a scalable solution, given the increasing customer base, which led to poor customer satisfaction
– Low borrower responsiveness
– Non-adherence to regulatory compliance
– Low borrower responsiveness
– Non-adherence to regulatory compliance
To address this, the company chose to employ Credgenics, a platform that uses ML to automate collection process. Here’s how the platform helped the company perform more efficiently.
- Categorized approach for tailored communication and better handling
- Segmentation based on parameters such as demographics, financial history, and payment behavior
- Ascertain the best suited mode, channel, and time of communication
- Digital channels such as voicebots, chatbots, SMS, WhatsApp, IVR and email prioritized for low and medium risk segments
- Human tele-calling prioritized for high and very high-risk segments
The impact:
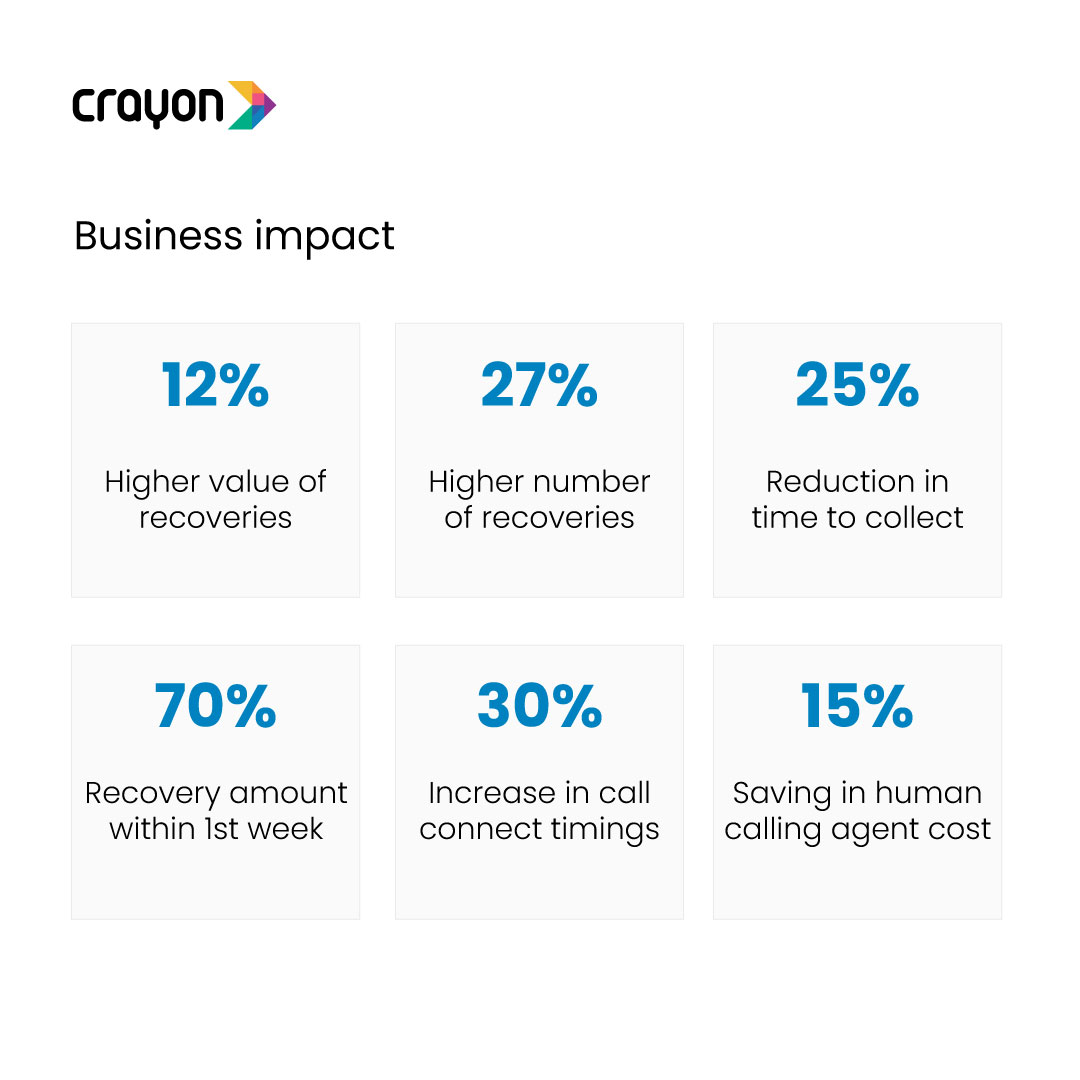
3. Personalized offers
AI’s analysis of a customer’s activities helps a lender pitch offers tailored for every customer. Along with improving customer experience, this also adds to customer loyalty. Better experience, better loyalty, more transaction volume.
In conclusion, AI is revolutionizing the lending industry by making credit scoring more accurate and inclusive. Traditional methods often fall short, but AI-driven models can use alternate data to provide fairer assessments, enabling access to financial support for more people. Lenders benefit from faster application evaluations, improved fraud detection, and personalized offers, enhancing efficiency and customer satisfaction. However, it’s essential to consider AI’s limitations, such as data security concerns, ethical issues in using personal data, and the challenge of ensuring data accuracy. Despite these challenges, the benefits of AI in lending—driving growth and fostering financial inclusion—are undeniable.
Ready to optimize your borrowers’ experience? Connect with our AI experts today!
In conclusion, AI is revolutionizing the lending industry by making credit scoring more accurate and inclusive. Traditional methods often fall short, but AI-driven models can use alternate data to provide fairer assessments, enabling access to financial support for more people. Lenders benefit from faster application evaluations, improved fraud detection, and personalized offers, enhancing efficiency and customer satisfaction. However, it’s essential to consider AI’s limitations, such as data security concerns, ethical issues in using personal data, and the challenge of ensuring data accuracy. Despite these challenges, the benefits of AI in lending—driving growth and fostering financial inclusion—are undeniable.
Ready to optimize your borrowers’ experience? Connect with our AI experts today!
Recent Blogs
Subscribe to the Crayon Blog. Get the latest posts in your inbox!