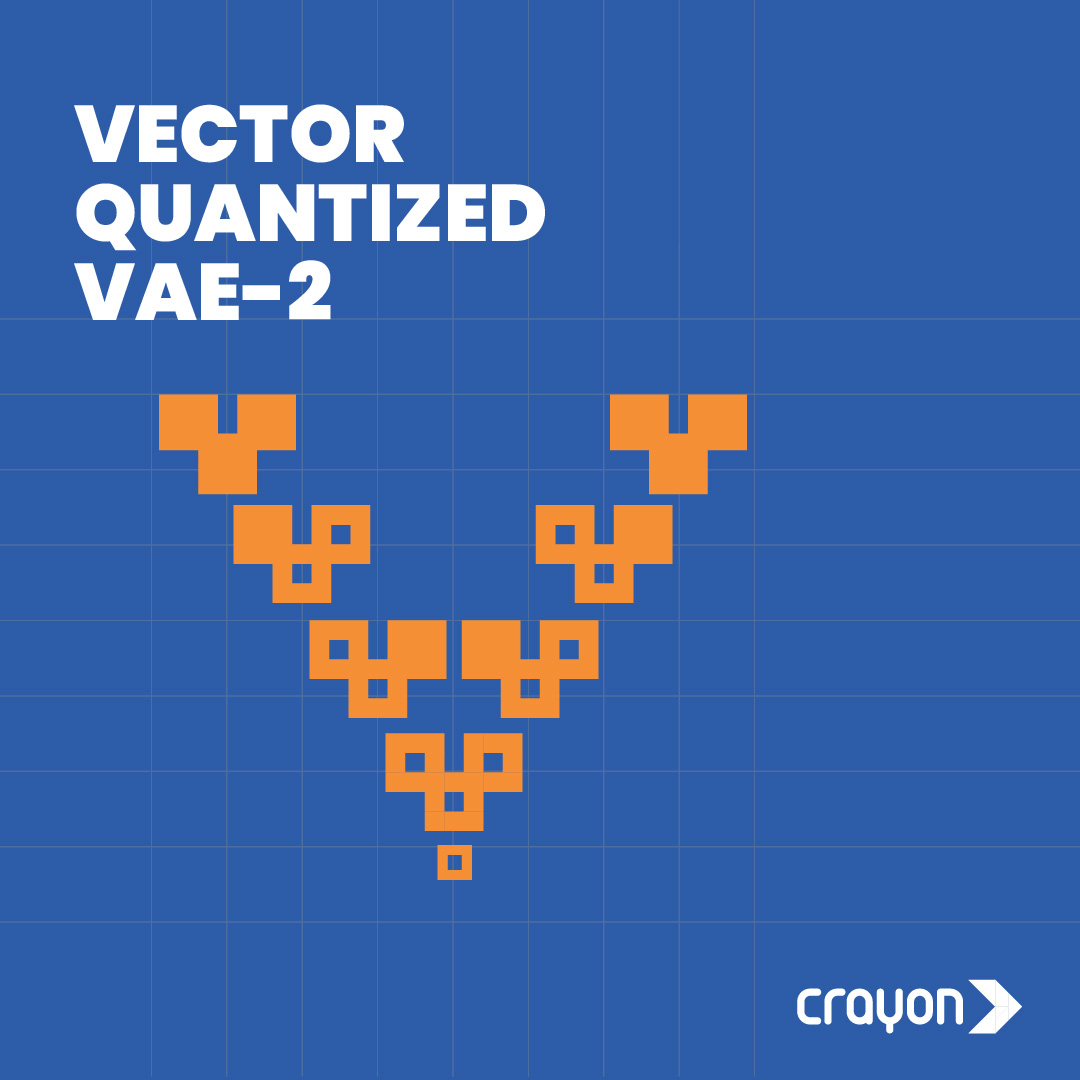
Vector Quantized
December 14, 2023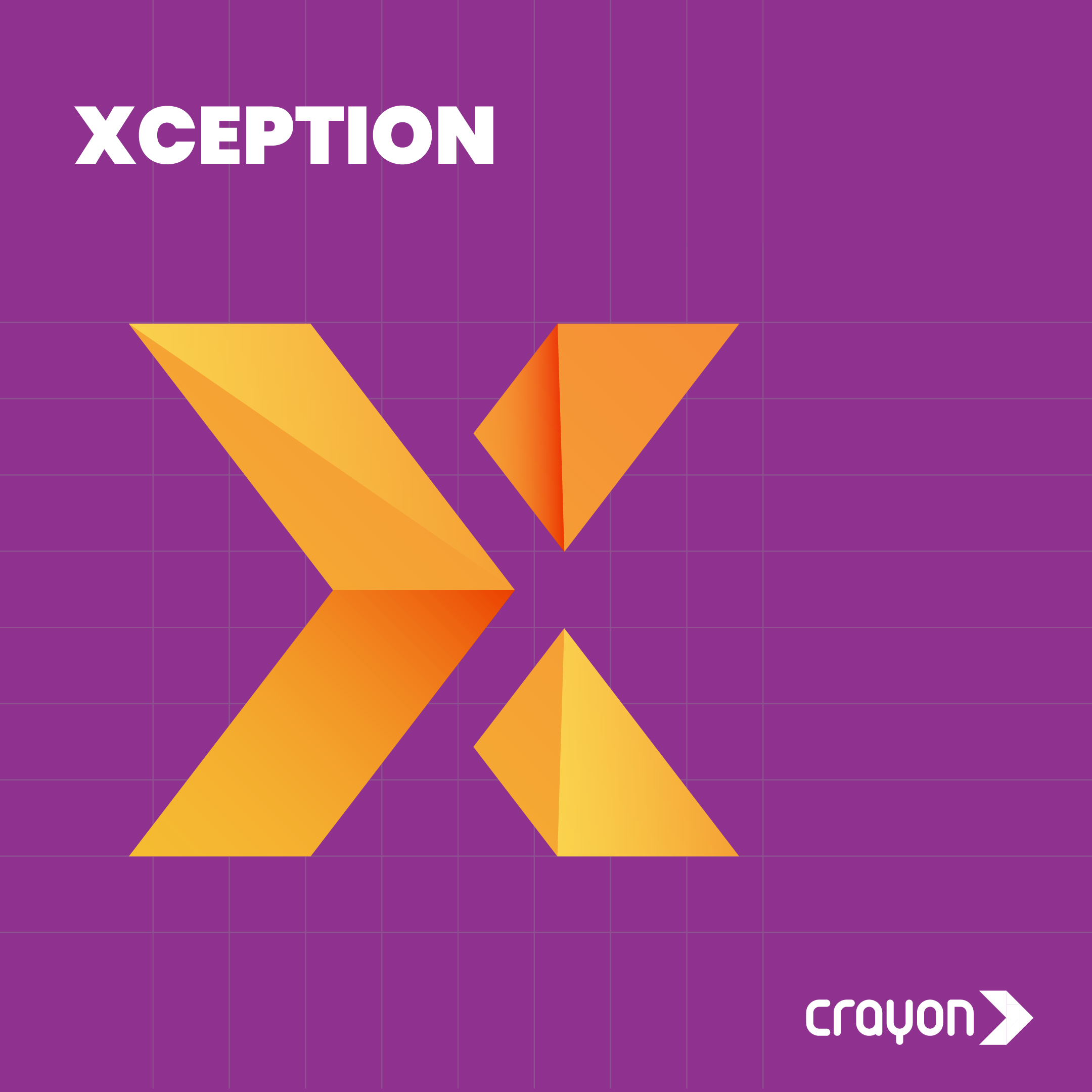
Xception
January 11, 2024#TheAIAlphabet
W for Winograd Schema Challenge
Published January 4, 2024 | Susanna Myrtle Lazarus
The Winograd Schema Challenge (WSC) is a linguistic benchmark designed to assess the capability of artificial intelligence systems to understand and generate human-like language in a nuanced context. Named after Terry Winograd, a computer scientist and professor, the challenge consists of a set of sentences that require common-sense reasoning and contextual understanding for accurate interpretation. Unlike traditional language processing tasks that may rely heavily on statistical patterns and predefined rules, the WSC demands a deeper comprehension of the subtleties inherent in human communication.
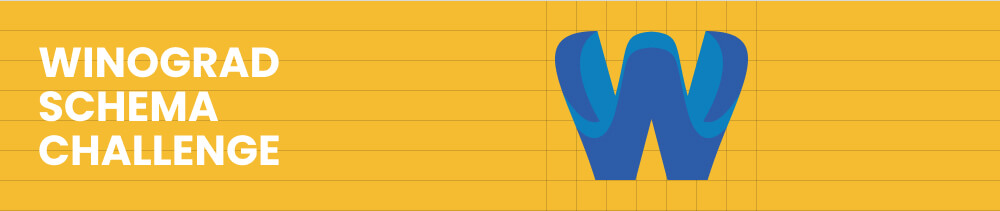
At its core, the challenge comprises pairs of sentences that differ only in a single word or phrase, often a pronoun. The task for the AI system is to correctly identify the referent of the ambiguous element, demonstrating an ability to grasp the underlying context and infer the intended meaning. These scenarios are carefully crafted to be resistant to simple pattern-matching techniques, requiring models to possess a more profound understanding of the world and its intricacies.
The WSC serves as a litmus test for natural language understanding, emphasizing the need for AI systems to move beyond mere linguistic surface-level processing. It aims to address the limitations of conventional language models that may excel in simpler tasks but falter when confronted with the complexities of real-world communication.
In contrast to the WSC, the Turing Olympics – a term not formally established but used here for comparison purposes – could refer to a broader set of challenges inspired by Alan Turing’s vision of machines exhibiting human-like intelligence. The Turing Test, proposed by Turing himself, focuses on a machine’s ability to indistinguishably converse with a human evaluator. While both the WSC and the Turing Test assess AI’s language capabilities, they differ in their approach and scope.
The WSC hones in on specific linguistic nuances, requiring models to navigate through context-dependent ambiguities. It delves into the intricacies of understanding pronouns and their referents, thereby targeting a more granular aspect of language comprehension. In contrast, the Turing Test encompasses a wider range of tasks, evaluating a machine’s overall ability to mimic human intelligence across diverse conversational topics without a specific emphasis on nuanced language understanding.
In essence, the WSC and the Turing Olympics complement each other in the evaluation of AI systems. While the former sharpens the focus on nuanced language comprehension, the latter encapsulates a holistic assessment of artificial intelligence, measuring its capacity to engage in human-like conversations across various domains. Together, these challenges contribute to the ongoing pursuit of AI that not only processes language but also interprets it with the depth and context-sensitivity inherent in human communication.
The WSC serves as a litmus test for natural language understanding, emphasizing the need for AI systems to move beyond mere linguistic surface-level processing. It aims to address the limitations of conventional language models that may excel in simpler tasks but falter when confronted with the complexities of real-world communication.
In contrast to the WSC, the Turing Olympics – a term not formally established but used here for comparison purposes – could refer to a broader set of challenges inspired by Alan Turing’s vision of machines exhibiting human-like intelligence. The Turing Test, proposed by Turing himself, focuses on a machine’s ability to indistinguishably converse with a human evaluator. While both the WSC and the Turing Test assess AI’s language capabilities, they differ in their approach and scope.
The WSC hones in on specific linguistic nuances, requiring models to navigate through context-dependent ambiguities. It delves into the intricacies of understanding pronouns and their referents, thereby targeting a more granular aspect of language comprehension. In contrast, the Turing Test encompasses a wider range of tasks, evaluating a machine’s overall ability to mimic human intelligence across diverse conversational topics without a specific emphasis on nuanced language understanding.
In essence, the WSC and the Turing Olympics complement each other in the evaluation of AI systems. While the former sharpens the focus on nuanced language comprehension, the latter encapsulates a holistic assessment of artificial intelligence, measuring its capacity to engage in human-like conversations across various domains. Together, these challenges contribute to the ongoing pursuit of AI that not only processes language but also interprets it with the depth and context-sensitivity inherent in human communication.
Recent Blogs
Subscribe to the Crayon Blog. Get the latest posts in your inbox!