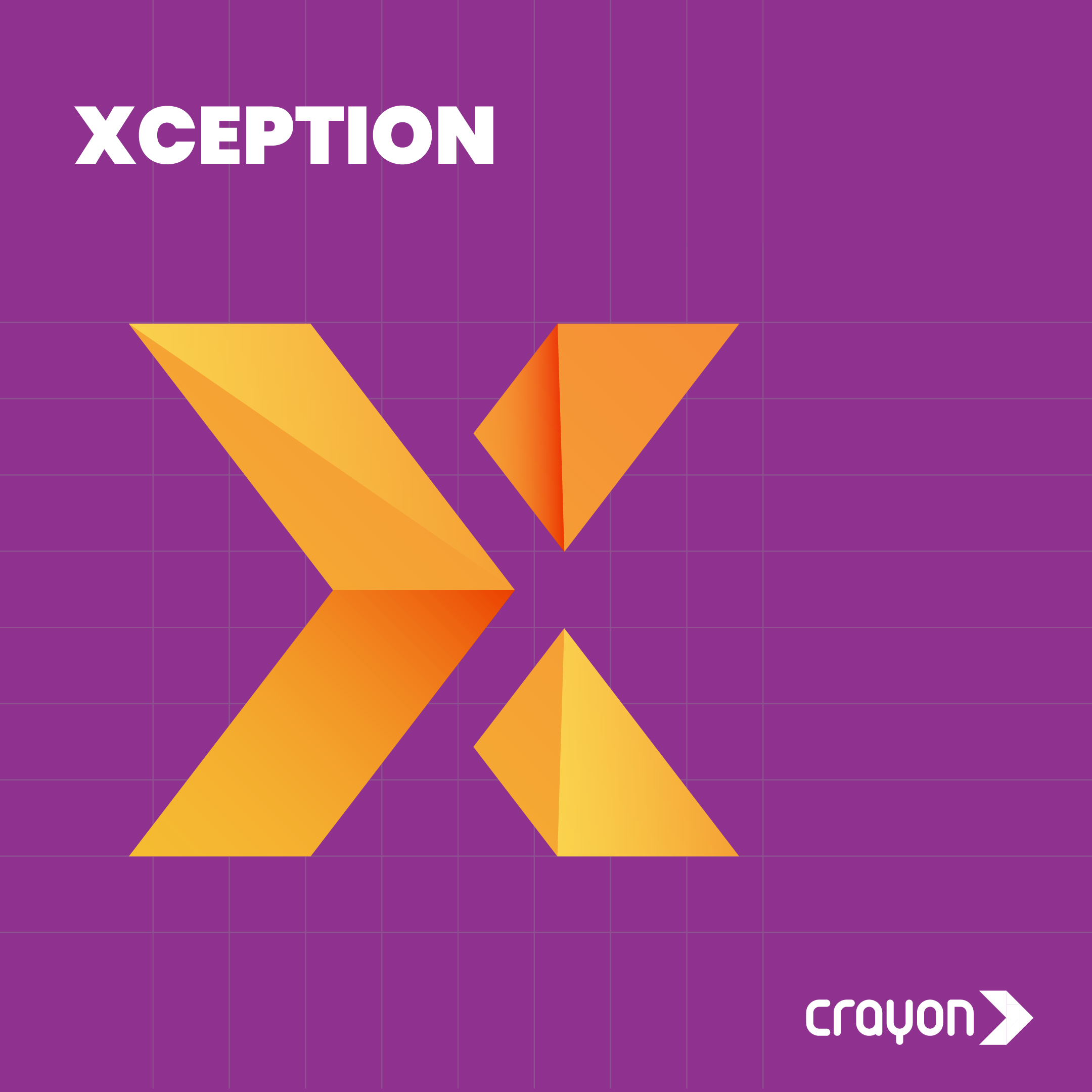
Xception
January 11, 2024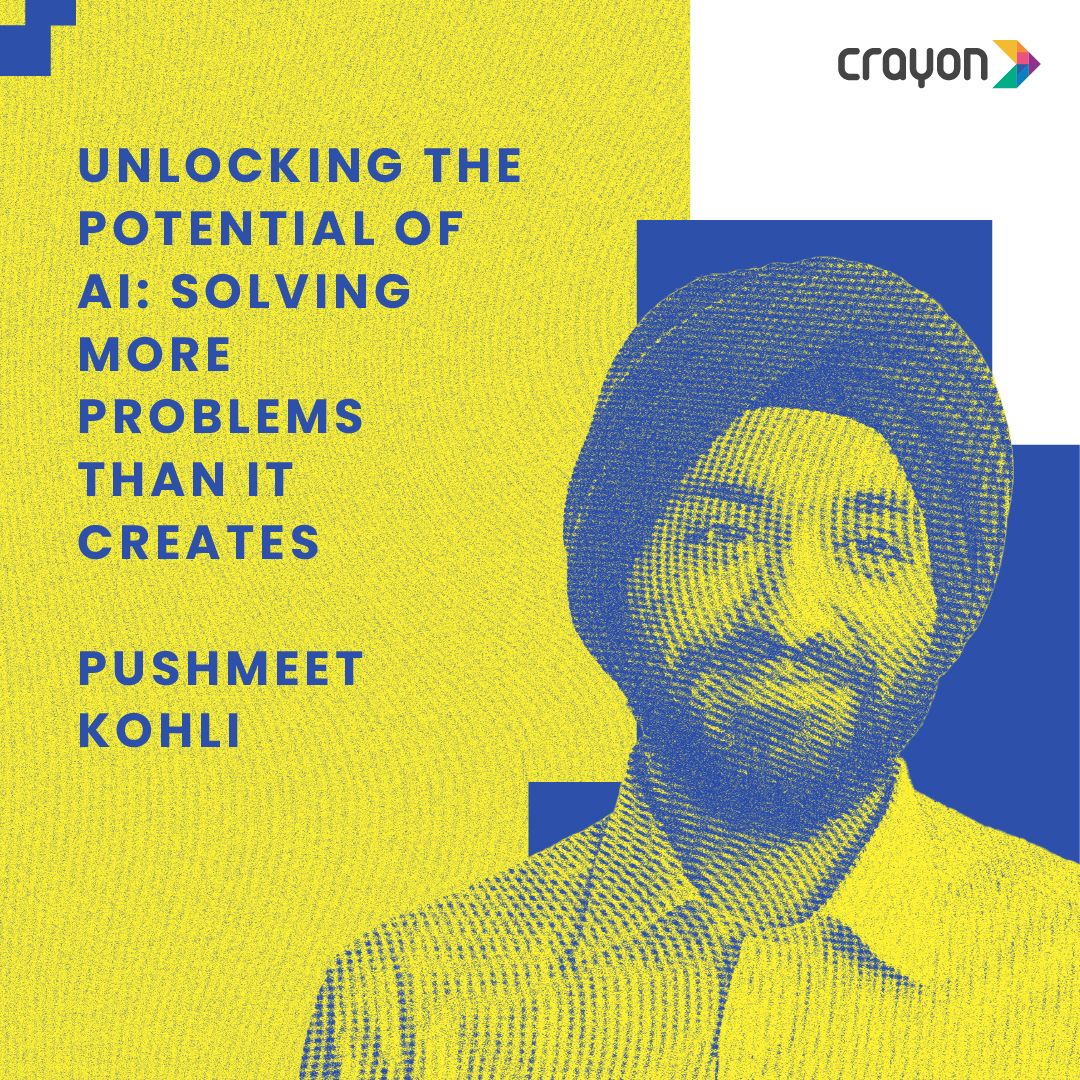
Legends of AI: Pushmeet Kohli
January 19, 2024#TheAIAlphabet
Y for YOLO
Published January 18, 2024 | Susanna Myrtle Lazarus
Imagine you’re at a massive art gallery filled with countless paintings, each depicting a variety of scenes and objects. Your task is to quickly identify and label everything in the artwork. Traditionally, this process involves meticulously examining each painting in multiple passes – first identifying regions of interest and then categorizing them. It’s akin to reading a book chapter by chapter.
Now, enter You Only Look Once (YOLO), a game-changing approach that transforms this process into a streamlined and efficient operation. YOLO treats object detection like reading the entire book at once. Instead of breaking down the artwork into sections and analyzing each independently, YOLO sweeps across the entire canvas, capturing the essence in a single view—a “you only look once” moment.
In practical terms, YOLO divides the artwork into a grid, much like a large puzzle. Each puzzle piece corresponds to a cell, and within each cell, YOLO predicts the boundaries of objects (like the edges of distinct shapes in a puzzle) and the likelihood of each object belonging to a particular category. This simultaneous prediction of bounding boxes and class probabilities for each cell constitutes the holistic output of the YOLO algorithm.
Now, enter You Only Look Once (YOLO), a game-changing approach that transforms this process into a streamlined and efficient operation. YOLO treats object detection like reading the entire book at once. Instead of breaking down the artwork into sections and analyzing each independently, YOLO sweeps across the entire canvas, capturing the essence in a single view—a “you only look once” moment.
In practical terms, YOLO divides the artwork into a grid, much like a large puzzle. Each puzzle piece corresponds to a cell, and within each cell, YOLO predicts the boundaries of objects (like the edges of distinct shapes in a puzzle) and the likelihood of each object belonging to a particular category. This simultaneous prediction of bounding boxes and class probabilities for each cell constitutes the holistic output of the YOLO algorithm.
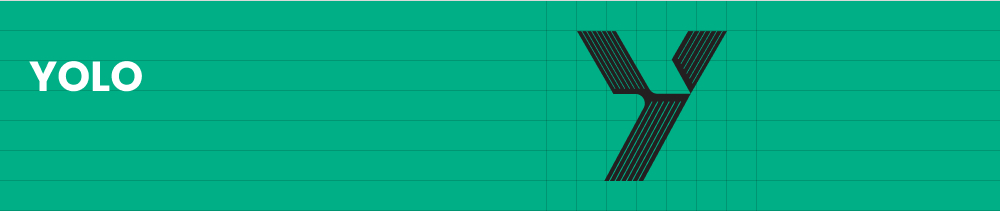
What makes YOLO truly stand out is its ability to process information in real-time. Picture this as flipping through the gallery’s paintings in rapid succession, absorbing the details immediately. Traditional methods may require a meticulous examination of each painting, one after the other, but YOLO’s single-pass approach significantly expedites the process—a game-changer for scenarios where speed is crucial, such as identifying objects in live video feeds or guiding autonomous vehicles through a dynamic environment.
Now, let’s extend the analogy to the adaptability of YOLO. Imagine the paintings in the gallery vary not only in content but also in size – some showcasing intricate details, while others display larger, more prominent subjects. YOLO, like a versatile viewer, excels at comprehensively assessing both small and large artworks simultaneously. It adjusts its focus seamlessly, predicting bounding boxes for various object sizes and ensuring a comprehensive understanding of the entire artistic landscape.
In essence, YOLO’s prowess lies in its ability to swiftly and comprehensively “read” an entire scene, much like a perceptive gallery visitor grasping the essence of each artwork with a single gaze. This approach has found applications in diverse fields, from swiftly recognizing objects in images and videos to enhancing real-time systems like surveillance and autonomous vehicles, where quick and accurate decision-making is paramount. Just as art evolves, so does YOLO, continuously shaping the landscape of AI-driven object detection.
Now, let’s extend the analogy to the adaptability of YOLO. Imagine the paintings in the gallery vary not only in content but also in size – some showcasing intricate details, while others display larger, more prominent subjects. YOLO, like a versatile viewer, excels at comprehensively assessing both small and large artworks simultaneously. It adjusts its focus seamlessly, predicting bounding boxes for various object sizes and ensuring a comprehensive understanding of the entire artistic landscape.
In essence, YOLO’s prowess lies in its ability to swiftly and comprehensively “read” an entire scene, much like a perceptive gallery visitor grasping the essence of each artwork with a single gaze. This approach has found applications in diverse fields, from swiftly recognizing objects in images and videos to enhancing real-time systems like surveillance and autonomous vehicles, where quick and accurate decision-making is paramount. Just as art evolves, so does YOLO, continuously shaping the landscape of AI-driven object detection.
Recent Blogs
Subscribe to the Crayon Blog. Get the latest posts in your inbox!