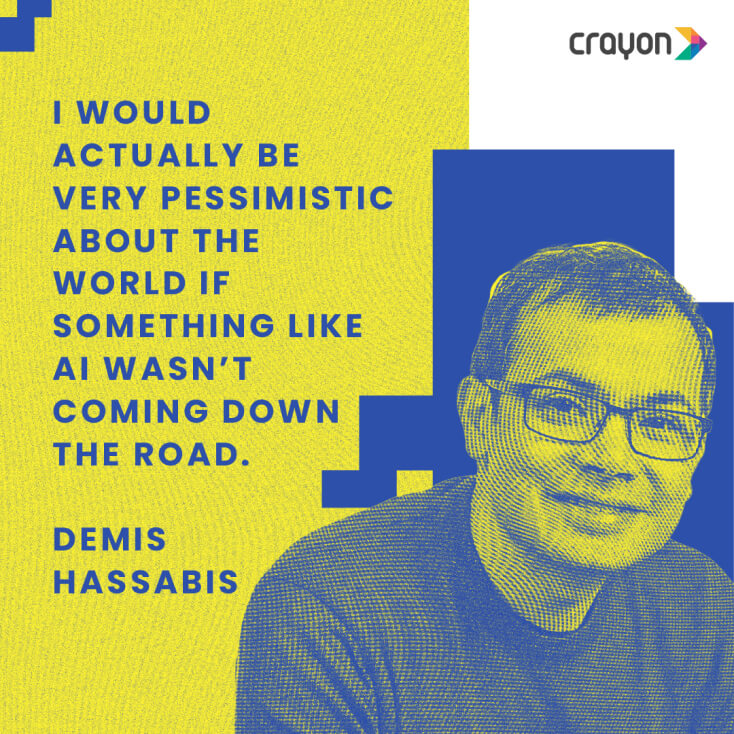
Legends of AI: Demis Hassabis
October 6, 2023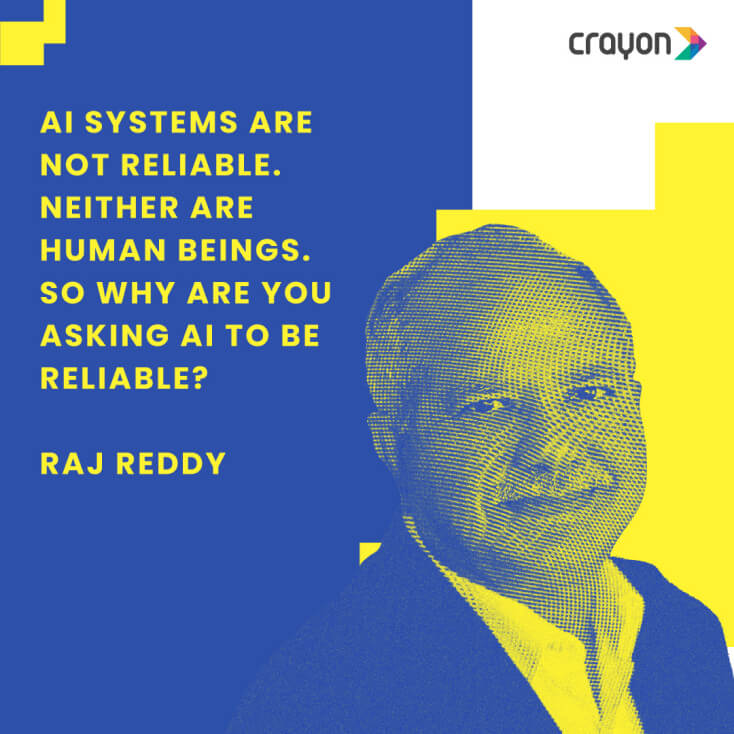
Legends of AI: Raj Reddy
October 13, 2023#TheAIAlphabet
N for Neurosymbolic Learning
Published October 12, 2023 | Susanna Myrtle Lazarus
How do you make a peanut butter and jelly sandwich? In front of you, you see bread, peanut butter, and jelly. You know what to do. But how do you know what to do next?
To put it simply, your brain combines the knowledge of what each ingredient is and how they interact to create a delicious sandwich. That’s kind of like what neurosymbolic learning does for artificial intelligence (AI)!
To put it simply, your brain combines the knowledge of what each ingredient is and how they interact to create a delicious sandwich. That’s kind of like what neurosymbolic learning does for artificial intelligence (AI)!
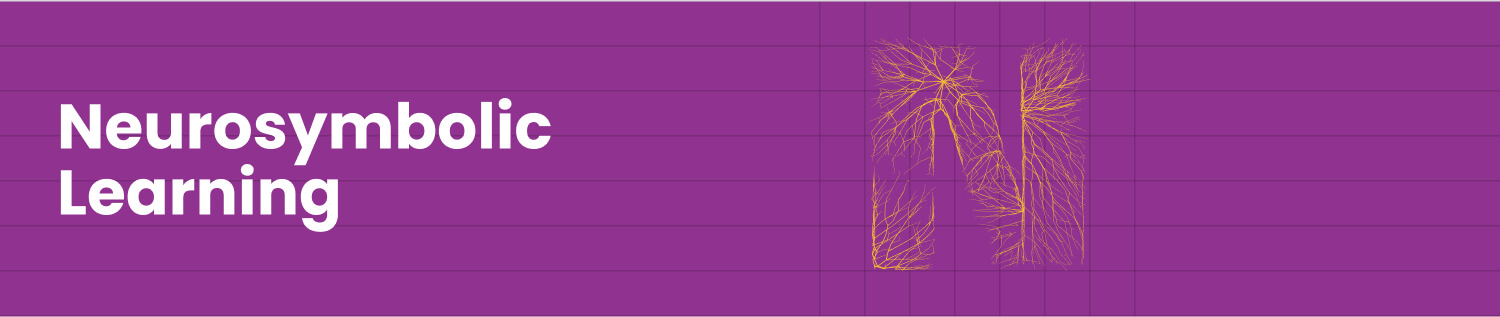
Neurosymbolic learning is a fancy term for a cool idea. It’s like blending two super-smart friends. The “neuro” part is the brainy neural networks, and the “symbolic” part is the logical, rule-following side. They team up to make AI smarter. The neural networks are like the creative, pattern-recognizing part, while the symbolic side is the rule-based, logical thinker.
Because neural networks are great at finding patterns in data, but they can be fooled by noise and irrelevant information. For example, a neural network trained to identify cats might also start identifying pictures of dogs as cats if the training data includes a lot of pictures of dogs.
Neurosymbolic learning combines the strengths of neural networks and symbolic reasoning to create a more robust and intelligent AI system. Symbolic reasoning is the ability to think about the world in terms of concepts and relationships. It’s what allows us to understand that a cat is different from a dog, even if they look similar.
In AI, this fusion of neural and symbolic thinking helps machines learn and understand the world in a more human-like way. It’s like giving a robot common sense! Neurosymbolic learning is still a relatively new field of research, but it has the potential to revolutionize many different areas of AI. Here are a few examples:
Because neural networks are great at finding patterns in data, but they can be fooled by noise and irrelevant information. For example, a neural network trained to identify cats might also start identifying pictures of dogs as cats if the training data includes a lot of pictures of dogs.
Neurosymbolic learning combines the strengths of neural networks and symbolic reasoning to create a more robust and intelligent AI system. Symbolic reasoning is the ability to think about the world in terms of concepts and relationships. It’s what allows us to understand that a cat is different from a dog, even if they look similar.
In AI, this fusion of neural and symbolic thinking helps machines learn and understand the world in a more human-like way. It’s like giving a robot common sense! Neurosymbolic learning is still a relatively new field of research, but it has the potential to revolutionize many different areas of AI. Here are a few examples:
Image recognition: Neurosymbolic learning can be used to improve the accuracy and robustness of image recognition systems. It could be trained to identify cats in pictures, even if the pictures are noisy or contain other animals.
Natural language processing: It can be used to improve the performance of machine translation and question answering. For example, it could be used to translate a text from one language to another, even if the text contains complex grammar or idiomatic expressions.
Reasoning and planning: It can develop AI systems that can reason and plan effectively. For instance, a robot that can navigate its way through a complex environment.
In essence, it’s like teaching AI to think with both its heart (neural networks) and its head (symbolic reasoning), which makes it much more capable and, well, sandwich-savvy in the world of artificial intelligence!
Natural language processing: It can be used to improve the performance of machine translation and question answering. For example, it could be used to translate a text from one language to another, even if the text contains complex grammar or idiomatic expressions.
Reasoning and planning: It can develop AI systems that can reason and plan effectively. For instance, a robot that can navigate its way through a complex environment.
In essence, it’s like teaching AI to think with both its heart (neural networks) and its head (symbolic reasoning), which makes it much more capable and, well, sandwich-savvy in the world of artificial intelligence!
Recent Blogs
Subscribe to the Crayon Blog. Get the latest posts in your inbox!