Here are the 10 key things to remember when you deal with big data.
1. More is better, may be not always!
When it comes to data, more is better, may be not always! Sometimes, “more” can be messy and too much irrelevant data makes it harder to get at what you’re looking for and can give you spurious signals. But “more data” means lower standard error, hence better model. Often, trends and anomalies, which communicate the most valuable information, can be seen only with more data (not with random sampling of data).
2. Data can be reused
Data is a raw material of all businesses and it does not expire after a single use. Data can be reused in unexpected ways and can provide answers to multiple problems at different times. Historic data set serves as an excellent example of how data can be reused and repurposed in ways unimaginable to the original collector of the data.
3. Data is an asset
Data is a valuable resource; it has real, measurable value. In simple terms, the purpose of data is to aid decision-making. Accurate, timely data is critical to accurate, timely decisions. So, data must be managed and protected carefully to obtain when and it is needed it.
5. Correlation does not imply causation
Correlation is not causation. Knowing the elusive cause of increased sales is a luxury but not a necessity to recreate the environment which furthered sales. Correlations can be identified more quickly and cheaply than root causes, and Big Data tells us what variables best predict if a customer will make a purchase. With this, businesses can increase or decrease variables to maximize output and sales. In Big Data, we become less concerned with the cause, which is often uncontrollable, and focus on the best correlations that predict a business phenomenon.
6. Value of data depends on its use
The value of big data lies in its use and reuse. Often, value is unleashed when very different datasets are combined to collectively answer big questions. With big data, the answer is more valuable than the sum of the data.
7. Big Data involves risks
As with all things, there is risk in big data. If information is power, then insights based on data from everywhere that predicts future behavior is the type of power found irresistible by archetypal villains (think Matrix). So let’s be clear, predictive analytics is based on numbers and how we interpret them. Results can be biased, numbers misleading and algorithms mis-analyzed. Understanding this and the inherent limitations of big data are crucial to mitigating risks.
8. Underestimating data quality
Data quality is a highly significant consideration. Poor quality can ruin analytics in any organization. For big data, overall data quality can degrade as unstructured and semistructured data are integrated into data sets. Improving data quality is an important consideration for processing big data. Without taking this step, the output often results in skewed results and can negatively impact the analytical systems in the enterprise.
9. Improperly contextualizing data
The fundamental logic behind processing textual data and executing text analytics lies with contextualization of the data. Without proper contextualization, the data can be processed with a lot of inaccuracy and produce skewed analytics.
10. Not grasping data complexity
Big data has multiple layers of hidden complexity that are not visible by simply inspecting it from an end-user perspective. The complexities are present in the data itself because of its structure and formats, content, and metadata. Without understanding the complexity, modeling a solution for the data set—whether statistical, mathematical, or text mining—can create erroneous results.
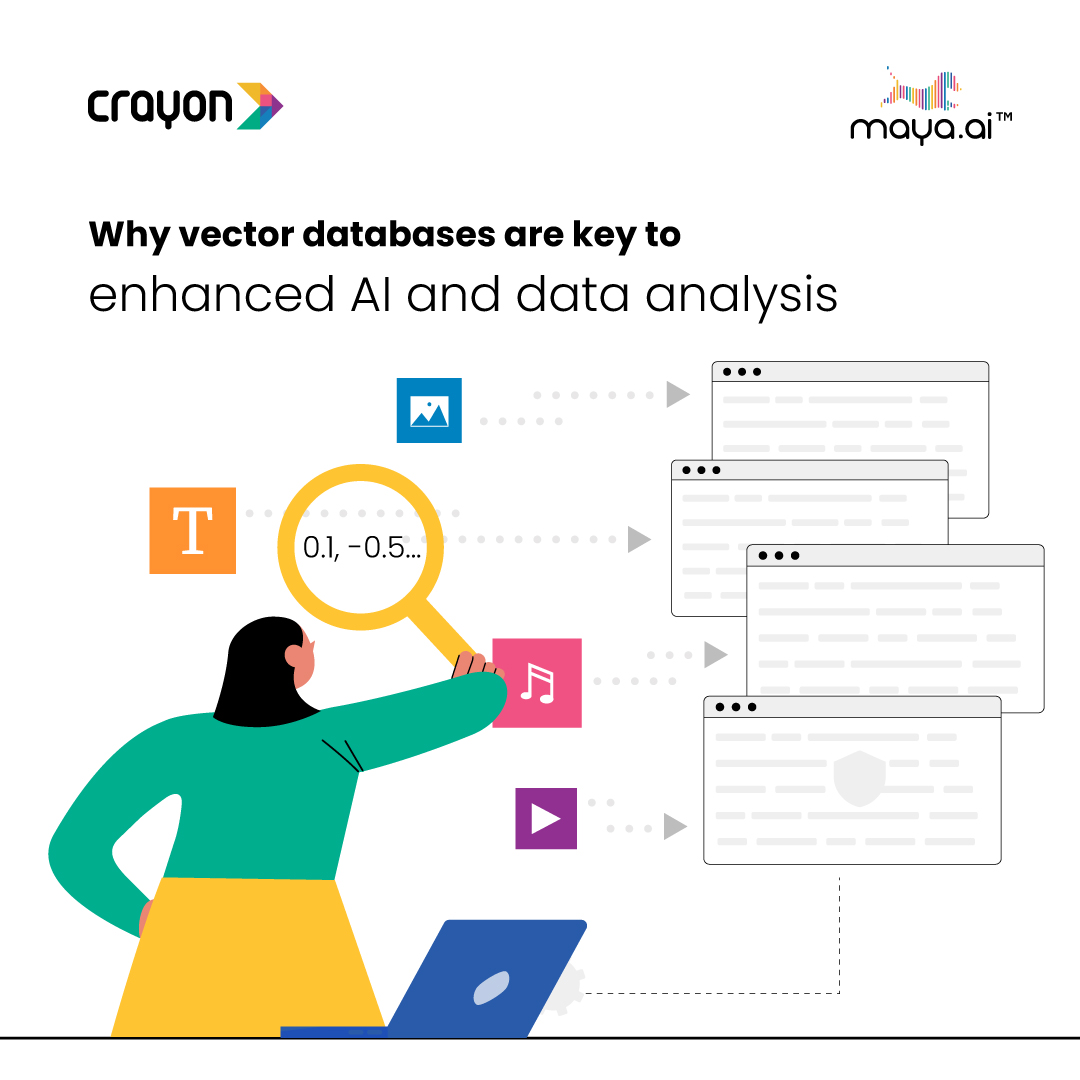
Why vector databases are key to enhanced AI and data analysis
In a...